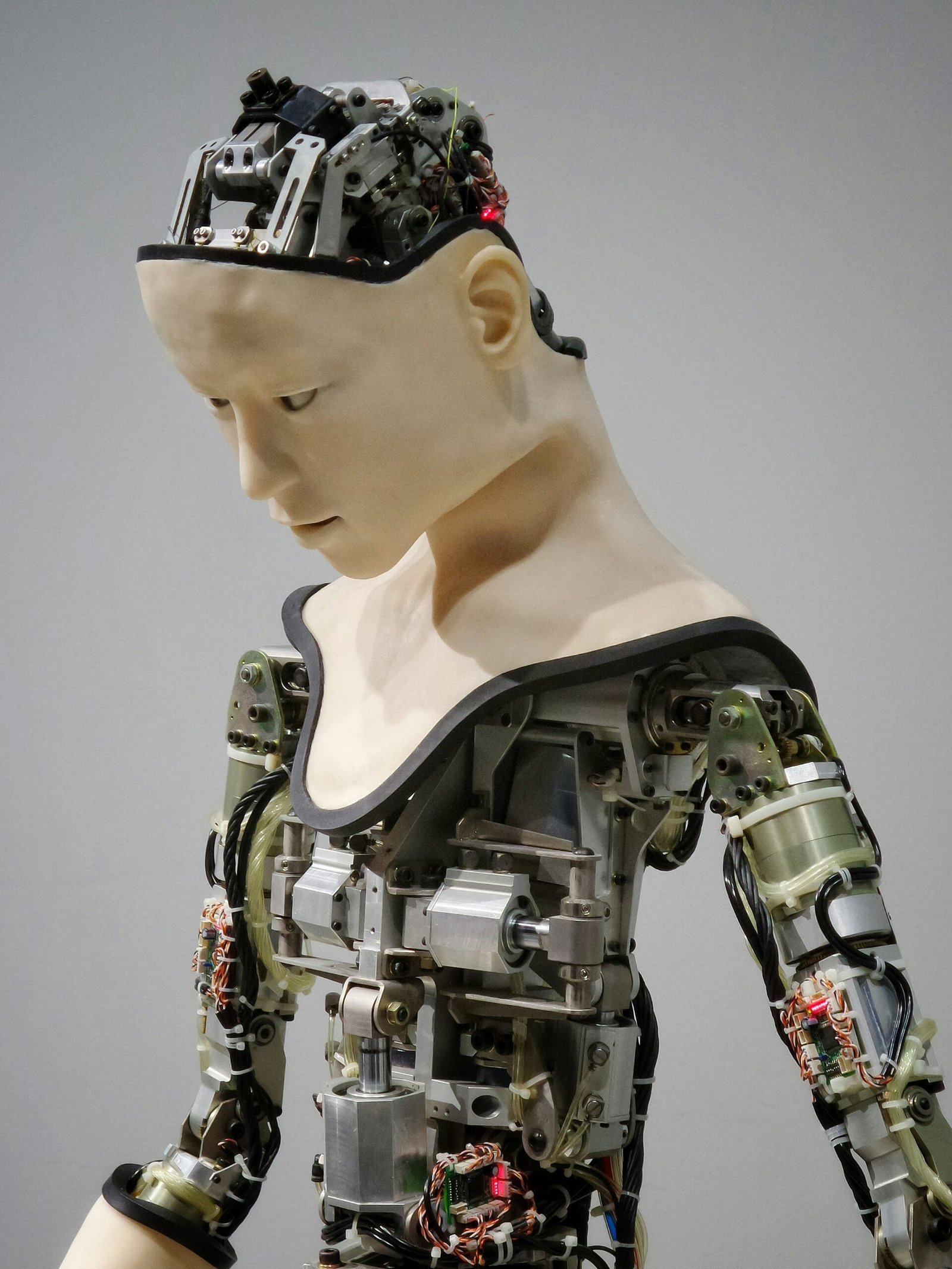
Understanding Artificial Intelligence and Machine Learning: Definitions and Key Concepts
Artificial Intelligence (AI) and Machine Learning (ML) are two intertwined fields that have fundamentally transformed various industries, from healthcare to finance. AI refers to the simulation of human intelligence processes by machines, particularly computer systems. These processes include learning, reasoning, and self-correction. ML, a subset of AI, involves the study of algorithms and statistical models that enable computers to perform tasks without explicit instructions, instead relying on patterns and inference.
The distinction between AI and ML is subtle but significant. AI encompasses a broader spectrum of technologies aimed at creating intelligent behavior in machines. ML specifically focuses on the mechanisms by which systems can learn from data. Key concepts within ML include supervised learning, unsupervised learning, and reinforcement learning. Supervised learning involves training a model on labeled data, where input-output pairs guide the learning process. In contrast, unsupervised learning deals with unlabeled data, allowing the system to infer the underlying structure. Reinforcement learning, another critical area, is based on rewarding desirable behaviors and penalizing undesired ones, akin to training a pet.
Neural networks and deep learning represent advanced techniques within ML. Neural networks, inspired by the human brain’s structure, consist of interconnected nodes or neurons that process information. Deep learning, a subset of neural networks, involves multiple layers of these neurons, enabling the analysis and interpretation of complex data patterns. This technology has powered significant advancements in image and speech recognition, natural language processing, and autonomous systems.
The historical evolution of AI and ML is marked by several milestones. The inception of AI can be traced back to the 1950s, with the Turing Test proposed by Alan Turing to evaluate machine intelligence. The 1980s saw the rise of neural networks, while the 2000s witnessed the emergence of big data and powerful computing resources, catalyzing the development of deep learning. Today, AI and ML continue to advance rapidly, driven by ongoing research and technological innovations.
Applications of AI and ML Across Various Industries
Artificial Intelligence (AI) and Machine Learning (ML) are revolutionizing numerous sectors by providing innovative solutions to complex problems and enhancing operational efficiencies. In the healthcare industry, AI and ML are pivotal in improving diagnostic accuracy and patient care. Technologies such as predictive analytics and image recognition are being employed to identify diseases at early stages and recommend personalized treatment plans. For example, AI algorithms can analyze medical images to detect anomalies like tumors, significantly aiding radiologists in their diagnostic processes.
In the finance sector, AI and ML are indispensable tools for fraud detection and risk management. Financial institutions utilize these technologies to scrutinize vast amounts of transaction data, identifying patterns indicative of fraudulent activities. Additionally, AI-driven algorithms facilitate automated trading and portfolio management, optimizing investment strategies based on real-time data analysis. By enhancing security and efficiency, AI is transforming the financial landscape.
The retail industry is also experiencing a paradigm shift with the integration of AI and ML. Personalized marketing and customer service are now more refined, thanks to these technologies. Retailers use AI to analyze customer behavior, preferences, and purchase history, enabling them to deliver tailored recommendations and offers. Chatbots powered by AI provide immediate customer support, enhancing the overall shopping experience.
Manufacturing is another domain where AI and ML are making significant contributions. Predictive maintenance, powered by machine learning algorithms, helps in anticipating equipment failures before they occur, thereby reducing downtime and maintenance costs. Furthermore, AI-driven robots and automation systems are streamlining production processes, ensuring higher precision and efficiency in manufacturing operations.
Transportation is rapidly evolving with the advent of autonomous vehicles, a direct result of advances in AI and ML. Self-driving cars, trucks, and drones are being developed and tested, promising to revolutionize logistics and reduce human error on the roads. AI systems in these vehicles process vast amounts of data from sensors and cameras in real-time, making split-second decisions to navigate safely and efficiently.
Overall, the transformative impact of AI and ML across various industries is undeniable. From enhancing business processes to improving customer experiences and operational efficiencies, these technologies are paving the way for a future replete with innovation and progress.
Challenges and Ethical Considerations in AI and ML
As artificial intelligence (AI) and machine learning (ML) technologies continue to evolve, they bring with them a host of challenges and ethical considerations that must be addressed. One of the primary concerns is data privacy. The vast amounts of data required to train AI and ML models often include sensitive personal information, raising significant privacy issues. Ensuring that data is collected, stored, and utilized in a manner that protects individual privacy is crucial. Implementing robust data anonymization techniques and strict data governance policies can help mitigate these concerns.
Another critical issue is the presence of bias in algorithms. AI and ML systems learn from historical data, which can contain biases that are inadvertently perpetuated by the models. This can lead to unfair or discriminatory outcomes, particularly in sensitive areas such as hiring, lending, and law enforcement. Addressing this challenge requires a proactive approach to identify and correct biases in data and algorithms. Techniques such as fairness-aware machine learning and the use of diverse datasets can contribute to more equitable AI outcomes.
The transparency of AI decision-making processes is also a significant ethical concern. Many AI systems operate as “black boxes,” making it difficult to understand how decisions are made. This lack of transparency can erode trust in AI technologies. Enhancing the interpretability and explainability of AI models is essential to promote accountability and build public trust. Methods like explainable AI (XAI) aim to provide insights into how AI systems arrive at their conclusions, making the decision-making process more transparent.
The potential for job displacement due to automation is another pressing issue. While AI and ML can drive efficiency and innovation, they can also lead to the displacement of workers in various industries. Addressing this challenge requires a multifaceted approach, including the development of reskilling and upskilling programs to prepare the workforce for the changing job landscape. Policymakers and industry leaders must collaborate to create strategies that balance technological advancement with employment stability.
To navigate these challenges, it is imperative to establish ethical guidelines and regulations governing the development and deployment of AI and ML technologies. These frameworks should emphasize the responsible use of AI, prioritizing human rights and societal well-being. Examples of current ethical dilemmas include the use of facial recognition technologies, which raise privacy and surveillance concerns, and autonomous vehicles, which pose questions about safety and liability. Potential solutions include the adoption of privacy-preserving technologies and the development of standards for AI safety and accountability.
The Future of AI and ML: Trends and Predictions
The landscape of Artificial Intelligence (AI) and Machine Learning (ML) is rapidly evolving, heralding a new era of technological advancements and innovations. One of the most promising technologies on the horizon is quantum computing. Quantum computers are expected to exponentially accelerate AI and ML processes, enabling the analysis of vast datasets and complex calculations with unprecedented speed and accuracy. This leap could revolutionize fields ranging from drug discovery to financial modeling, making previously intractable problems solvable.
Advanced neural networks are another key area of development. These networks, often referred to as deep learning systems, are becoming increasingly sophisticated. They are capable of mimicking human cognitive functions such as vision, speech recognition, and decision-making. As these networks evolve, they are expected to enhance the capabilities of AI, making it more intuitive and adaptable. This evolution will likely lead to more personalized and efficient AI applications in areas such as healthcare, education, and customer service.
AI-driven cybersecurity is also gaining traction. As cyber threats become more sophisticated, traditional security measures can fall short. AI and ML can proactively identify and mitigate these threats by analyzing patterns and predicting potential breaches. This capability is crucial for protecting sensitive data and maintaining trust in digital systems across various sectors, including finance, healthcare, and government.
The societal impact of AI and ML cannot be overstated. These technologies are poised to transform the global workforce by automating routine tasks and augmenting human capabilities. While this shift may lead to job displacement in certain sectors, it also promises to create new opportunities and industries, necessitating a rethinking of education and skill development to prepare the workforce for an AI-driven future.
Moreover, AI and ML are increasingly being harnessed to address pressing global challenges. In the realm of climate change, AI can optimize energy consumption, monitor environmental changes, and develop sustainable solutions. In public health, AI and ML can enhance disease prediction, improve diagnostic accuracy, and streamline the development of treatments. The integration of these technologies in addressing such challenges underlines their potential to contribute significantly to global well-being.